Applicable Fields
Materials Science, Solid-State Physics, Inorganic Chemistry, Chemical Physics, etc. Especially, Organic Chemistry and Biological Physics are out of scope.
Materials Discovery
Using the app integrated with the AI technology based on our research, you can conduct materials screening and may discover candidate compounds. Don't hesitate use the app to facilitate your frontier research.
Confidence
Every compound is given a predictive confidence according to its predictive variance. You can easily determine how reliable the system prediction is, taking a look at the confidence chart.
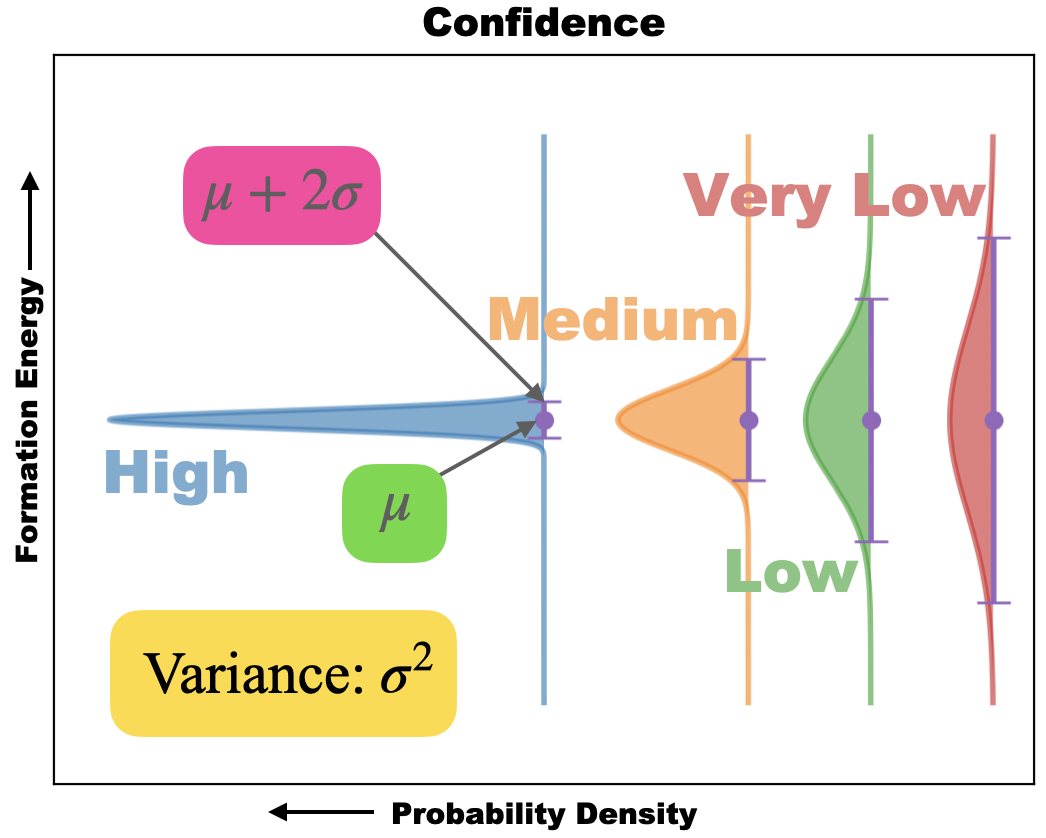
Customizable AI
You can increase the ensemble size of the machine learning model to make formation energy predictions more accurate, and the prototype database scale to gain more insight into the chemical system. The variance threshold is adjustable, so you can control the number of very low confident predictions.
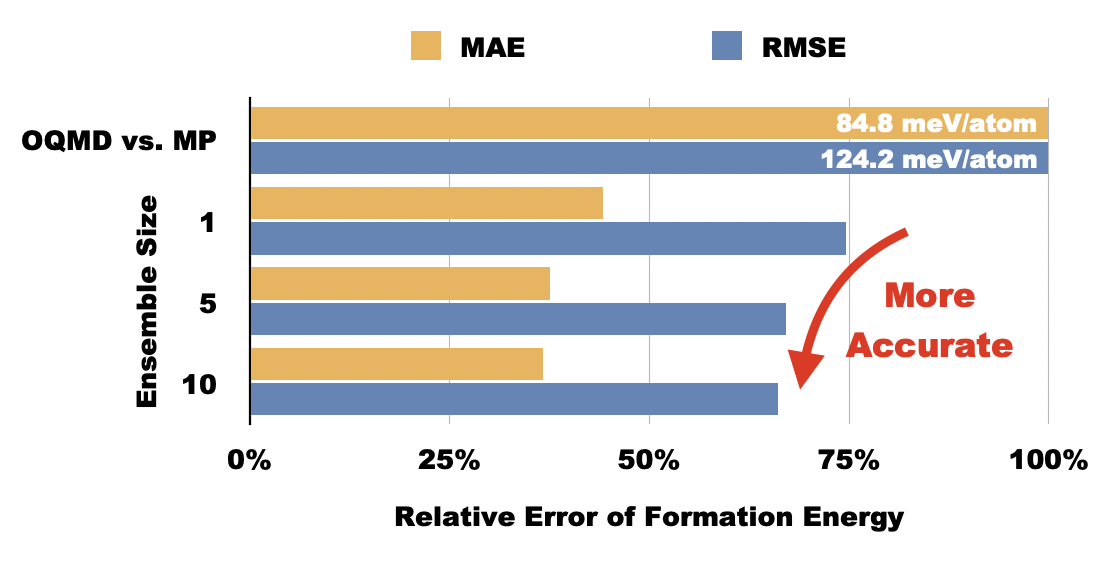
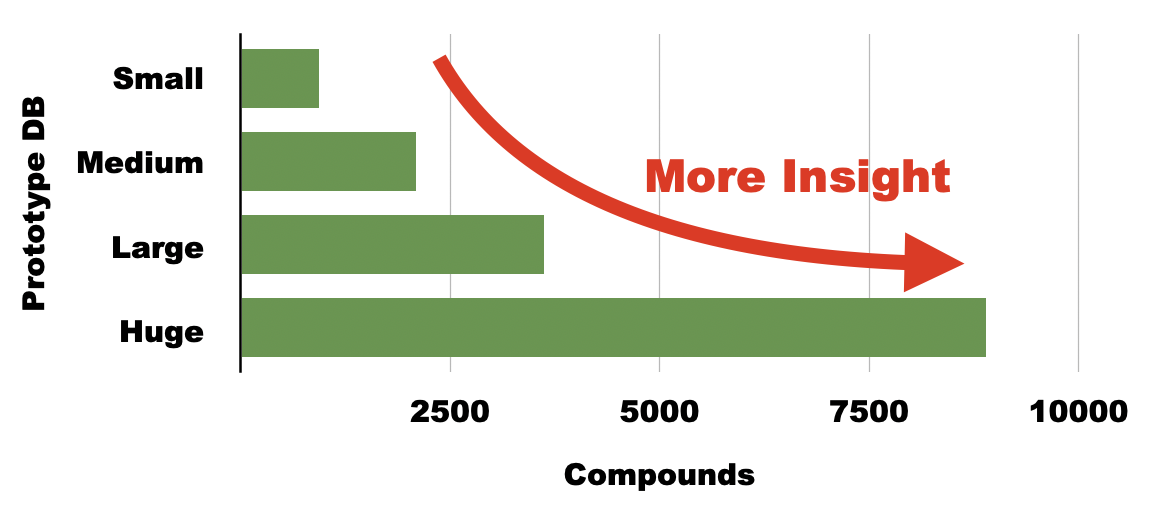
You can learn more about the AI technology used in the app reading the in-app documents and the reference papers.
On-Device Inference
The app uses no external resources to make a system prediction on your device. You can watch the phase diagram redrawn at the end of each stage.
Performace
Thanks to the efficient graph neural network architecture, the optimized graph generator, and the scale-invariant crystal graphs, the system prediction takes only a few seconds to minutes depending on the creation settings and your device. Moreover, optional multithreading accelerates the core of the AI tool - for example, on M1 iPad Air, the most computationally intensive tasks are sped up around 2.5x and take only about a minute.
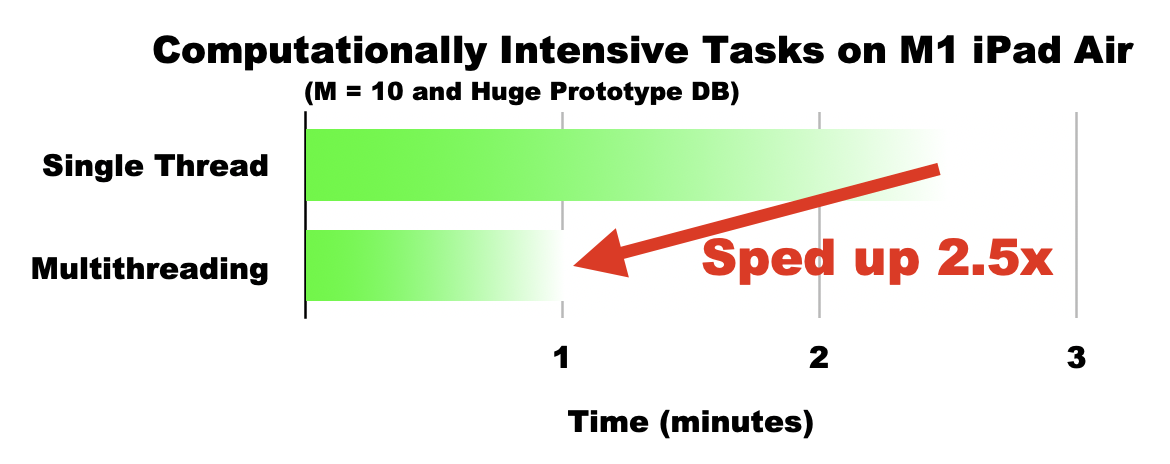
Security and Privacy
The app is a security-first AI tool for scientists. We don't track your activity in the app nor collect your data from the app. Your privacy therefore is maintained while you use the app. More info at the app's Privacy Policy.
Requirements
The app requires iOS (iPadOS) 17.2 or later and a device with the A12 Bionic chip or later.
Get iCrucible on the App Store now and see how helpful the AI app is.
Tips
Compound Screening
Adjust the stability threshold slider in the tool sheet to mark stable and metastable compounds with red-filled circles.
Overall Confidence
Tap the chart icon in the bottom bar to take a look at the confidence chart. You can determine how reliable the system prediction is.
Compound Table
Tap the table icon in the bottom bar to see the list of the stable and metastable compounds whose stability is lower than the stability threshold. They are sorted in increasing order by stability. You can easily pick up candidate compuounds from the table.
Preferences
Tap the settings icon to change default values for the phase diagram drawing. Especially, the initial mark size may be too small.
Support
If you have any questions or need help, please see the app's Support page.
iCrucible
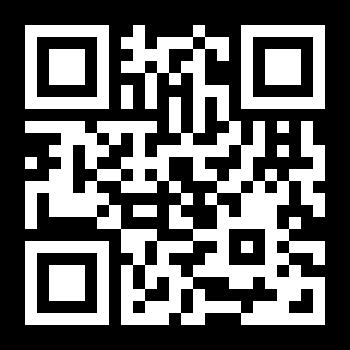
Research
Crystal Graph Gallery
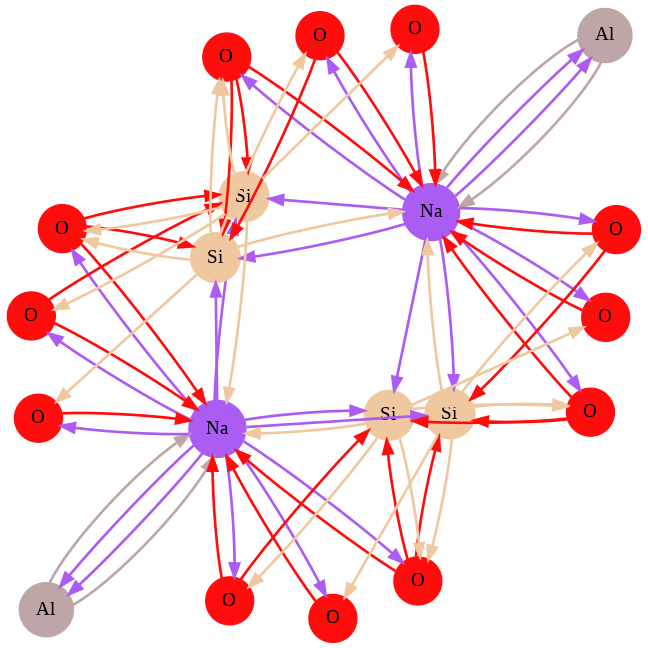
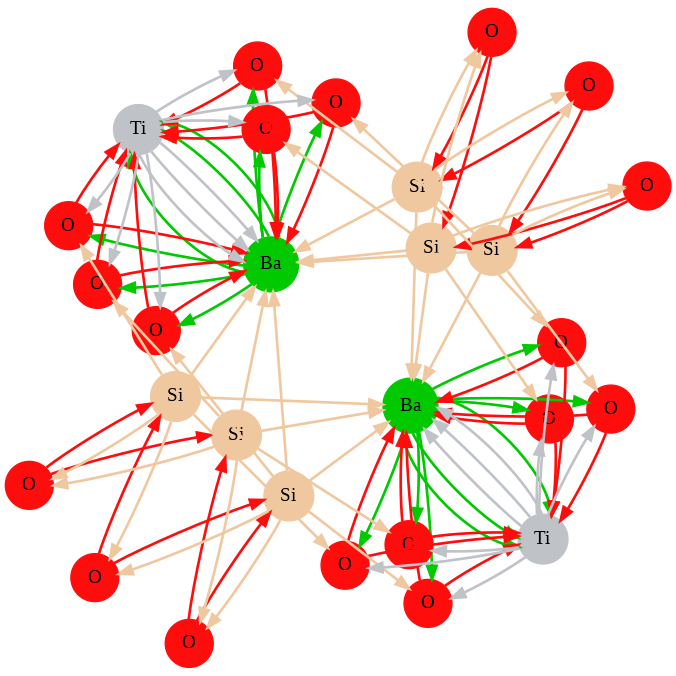
Crystal Graph Neural Networks for Data Mining in Materials Science
Abstract
Machine learning methods have been employed for materials prediction in various ways. It has recently been proposed that a crystalline material is represented by a multigraph called a crystal graph. Convolutional neural networks adapted to those graphs have successfully predicted bulk properties of materials with the use of equilibrium bond distances as spatial information. An investigation into graph neural networks for small molecules has recently shown that the no distance model performs almost as well as the distance model. This paper proposes crystal graph neural networks (CGNNs) that use no bond distances, and introduces a scale-invariant graph coordinator that makes up crystal graphs for the CGNN models to be trained on the dataset based on a theoretical materials database. The CGNN models predict the bulk properties such as formation energy, unit cell volume, band gap, and total magnetization for every testing material, and the average errors are less than the corresponding ones of the database. The predicted band gaps and total magnetizations are used for the metal-insulator and nonmagnet-magnet binary classifications, which result in success. This paper presents discussions about high- throughput screening of candidate materials with the use of the predicted formation energies, and also about the future progress of materials data mining on the basis of the CGNN architectures.(Published 27 May 2019)
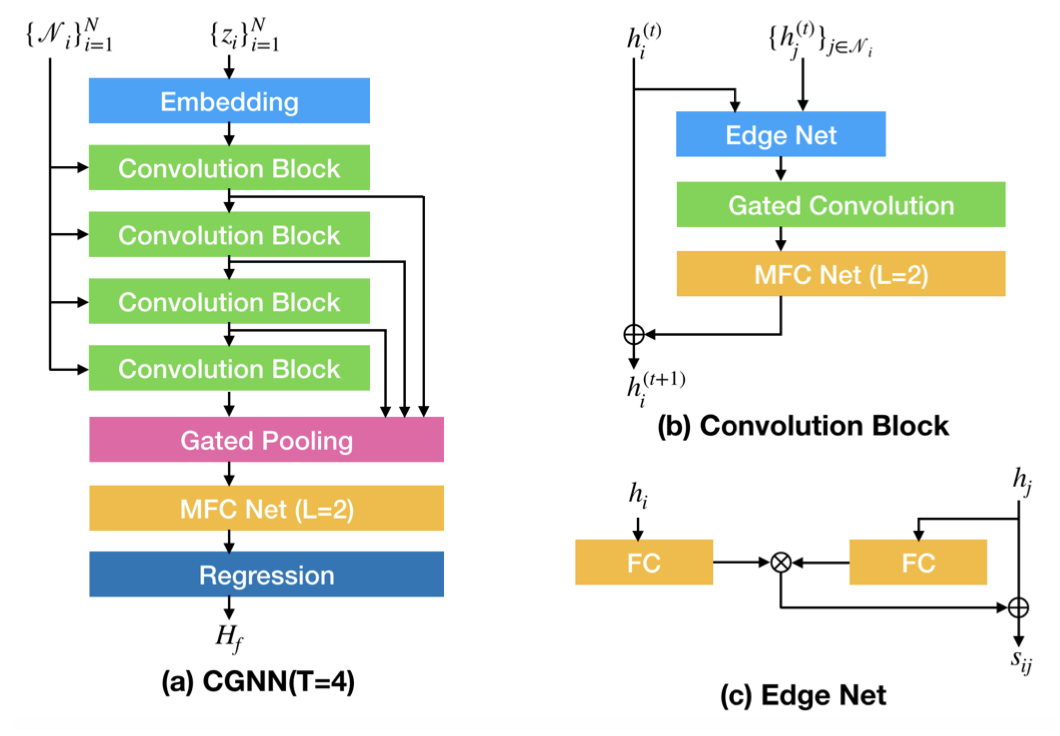
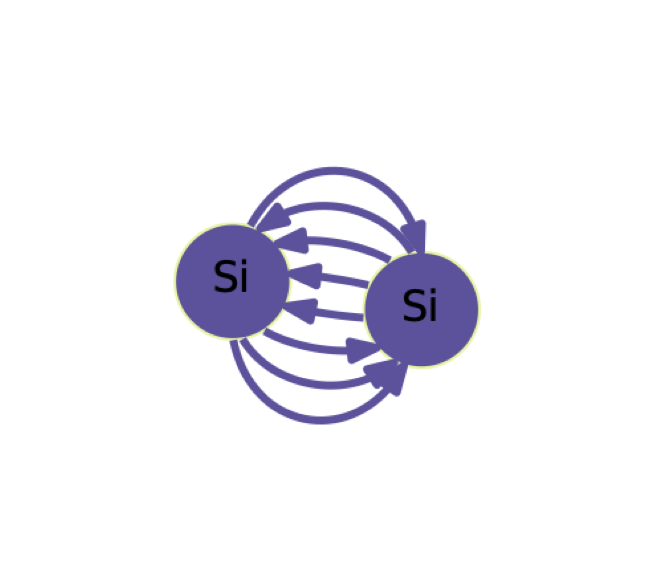
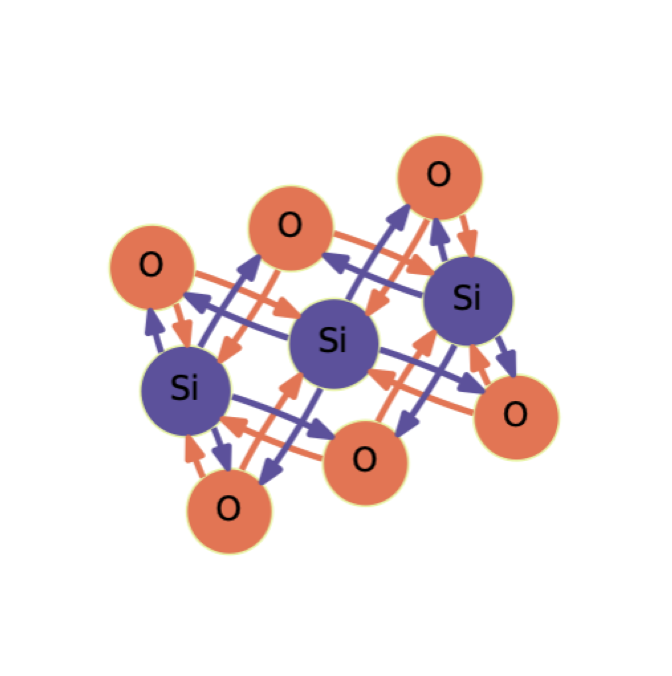
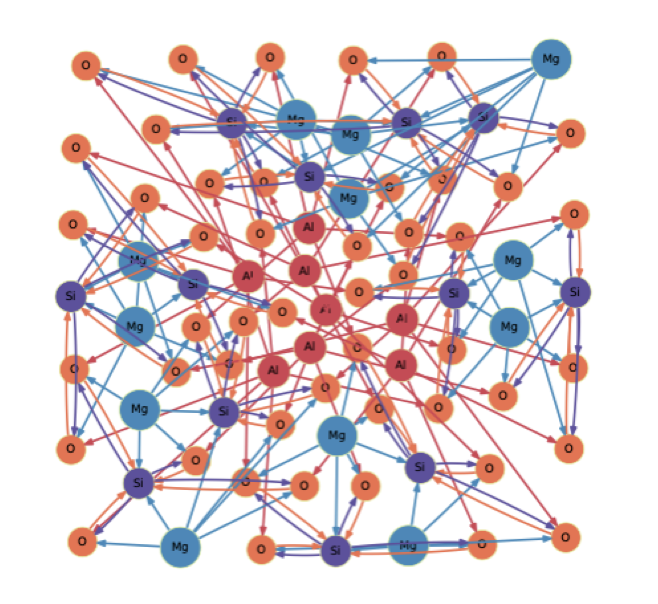
2_garnet.png)
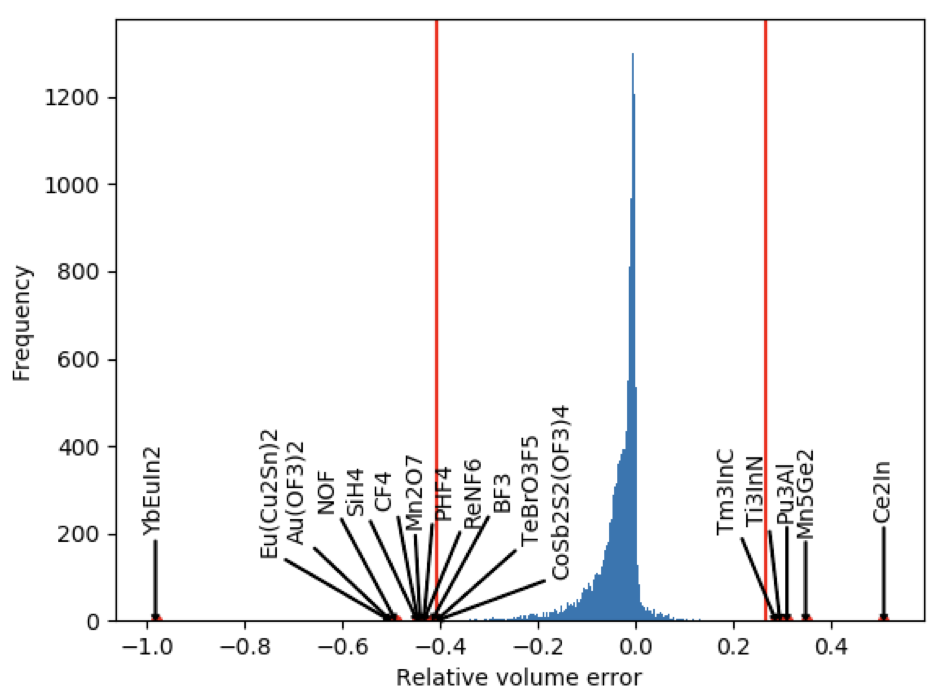
OQM9HK: A Large-Scale Graph Dataset for Machine Learning in Materials Science
Abstract
We introduce a large-scale dataset of quantum-mechanically calculated properties of crystalline materials for graph representation learning that contains approximately 900k entries (OQM9HK). This dataset is constructed on the basis of the Open Quantum Materials Database (OQMD) v1.5 containing more than one million entries, and is the successor to the OQMD v1.2 dataset containing approximately 600k entries (OQM6HK). We develop the graph creation algorithm to produce a binary edge-labeled (BEL) graph representing a crystalline material. The BEL graph has higher representability of crystal structure than the edge-unlabeled ones. In materials property prediction tasks, crystal graph neural networks trained on the BEL graph dataset perform better than ones on the other graph datasets. The OQM9HK graph dataset is available at the Zenodo repository, here.
(Published 30 September 2022, Updated 9 October 2022)
First version
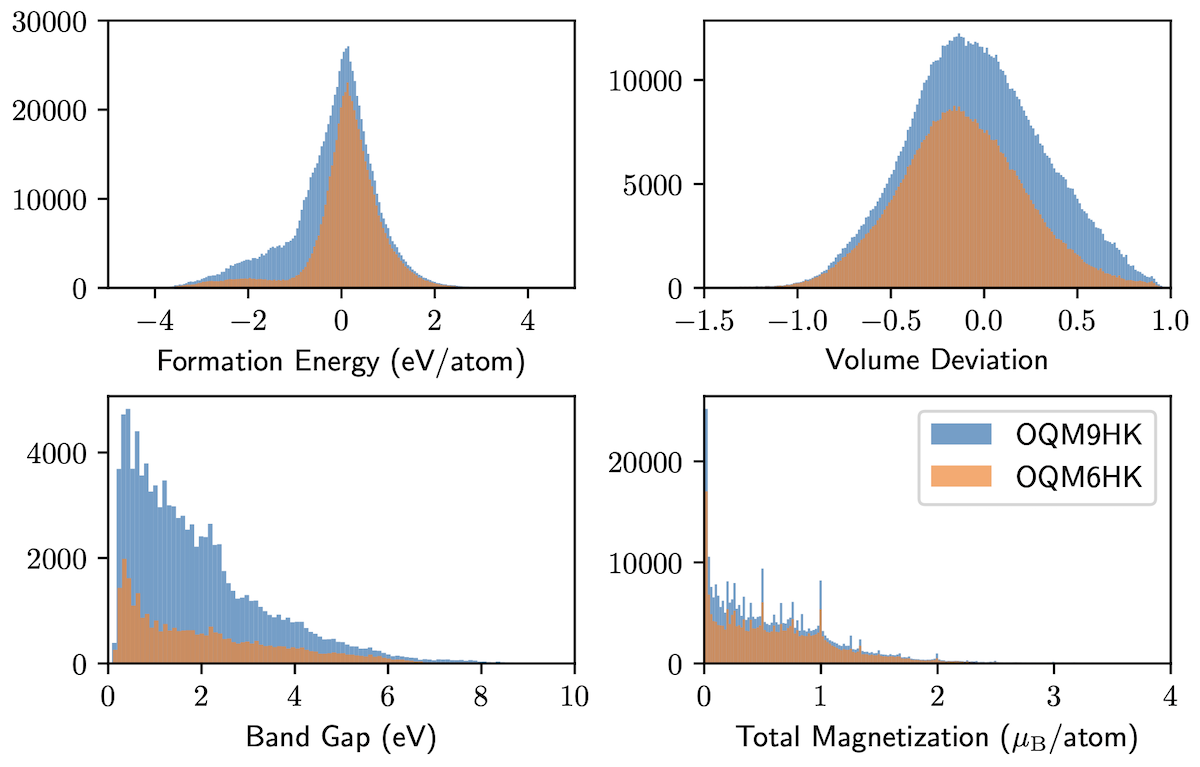
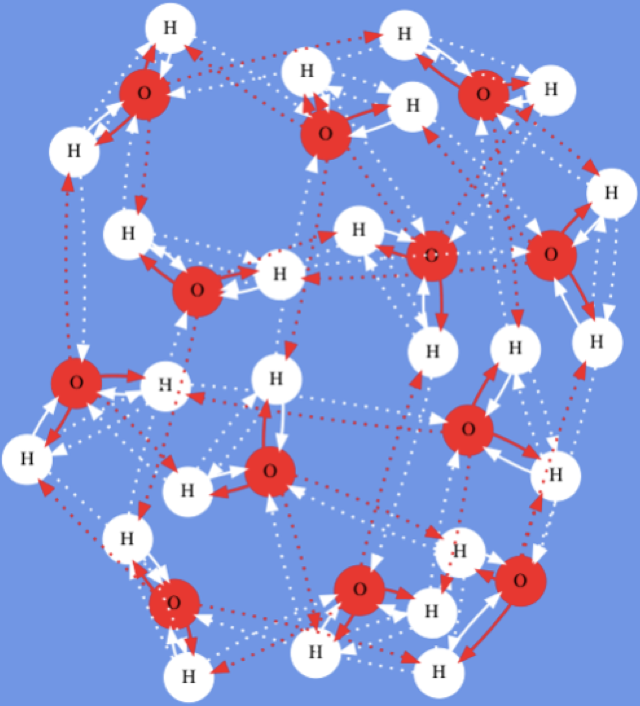
2_garnet.png)
2_garnet.png)
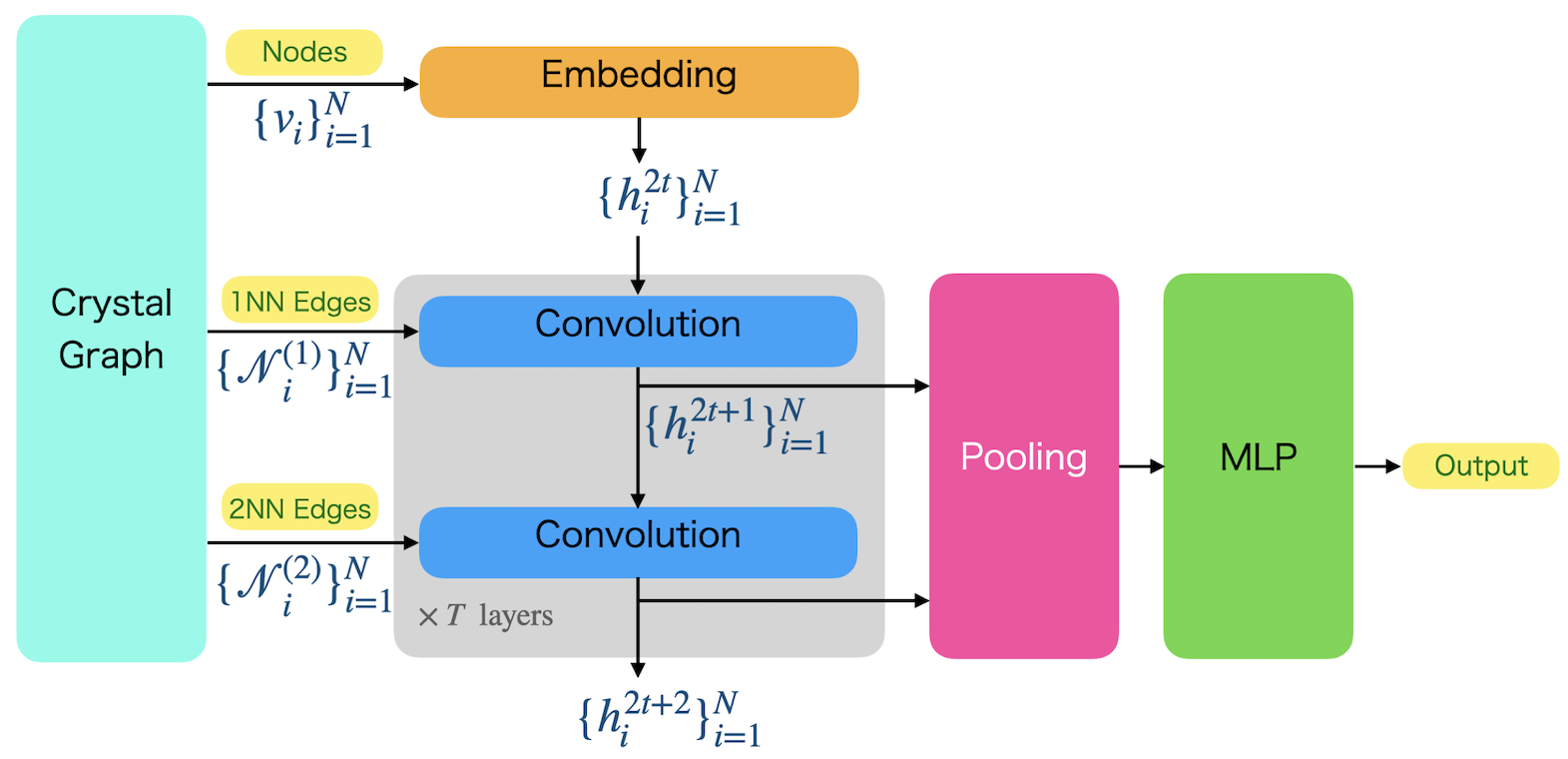
About Us
We are a research-oriented company to develop fast, efficient, and innovative solutions for scientific problems with mathematical concepts and computational technology.
Our areas of focus:
- Data Science
- Machine Learning
- Artificial Neural Networks
- Artificial Intelligence
- On-Device AI
iCrucible, an AI app for materials science, is an innovative product based on our recent research. Learn more
Takenori Yamamoto (aka Tony Y.), the founder and CEO of RIMCS LLC, is an expert in both materials science and machine learning. In 2018, he won first place in a machine learning competition about materials science on Kaggle, organized by NOMAD (as shown in Leaderboard). The first-place winning solution employs an n-gram approach to extract features from crystal graphs, whose details are described in the collaborative paper with NOMAD and the second- and third-place winner. The event is the origin of our recent research and the AI app, iCrucicle. His earlier scientific works can be found, for example, at ResearchGate. Our company was founded in 2014 and is located in Yokohama, Japan, a city of bayside. The corporate name is 合同会社数理計算科学総合研究所 in Japanese.
On the App Store, the seller of iCrucible is shown as "SURI KEISAN KAGAKU SOGO KENKYUSHO, LIMITED LIABILITY CO." It is another name for our company, named after the pronouncification in Japanese.
Contact Us
If you have any questions about our company, feel free to get in touch with us at info@rimcs.co.jp.